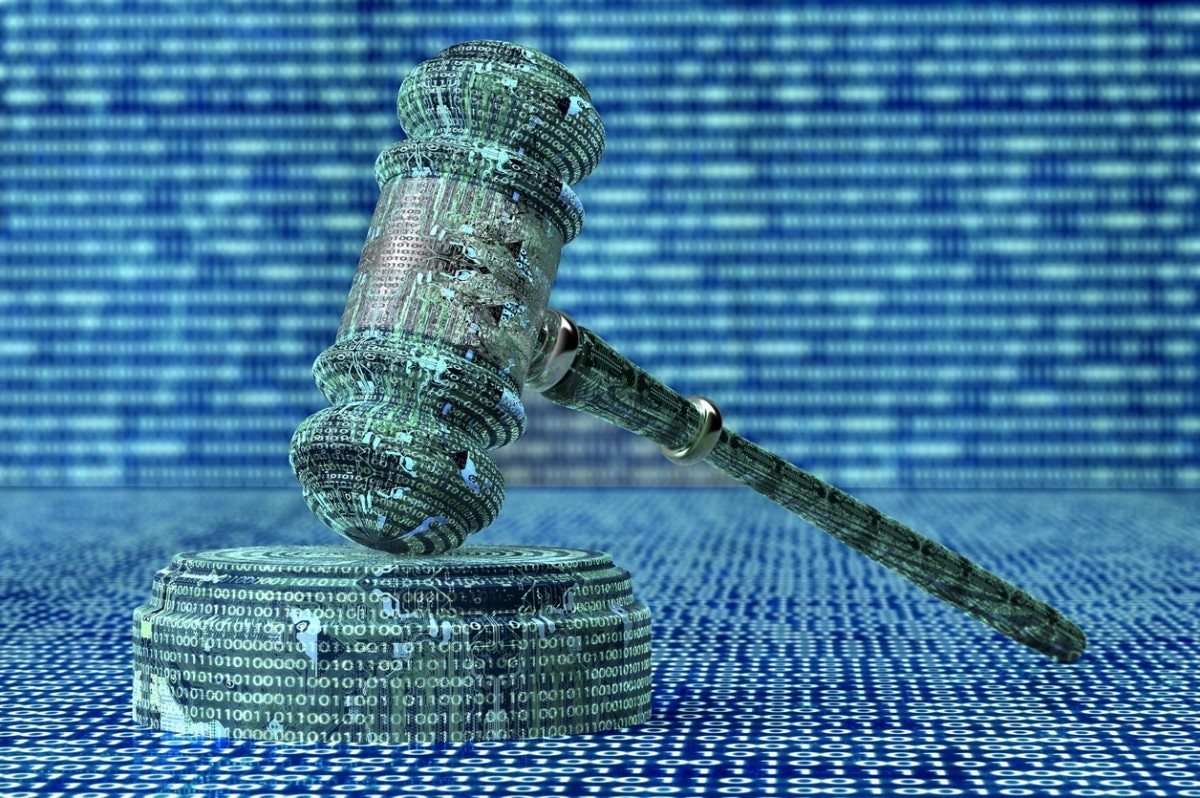
Eliminating lawsuits, fraud, and failure: Embracing AI risk management
“If it’s predictable, it’s preventable.”
This is the time-tested axiom of risk management that holds true across business verticals, from insurance and banking to healthcare and manufacturing. If you can identify risk by predicting failure, you can, to a large extent, prevent undesirable outcomes such as litigation, fraud, and lost revenue.
This idea has traditionally been enforced by deploying large teams specialized in analyzing risk across work practices and processes, claim histories, banking trends, and even machine maintenance and failure rates. But while the use of expert manpower is no doubt effective in implementing a risk management strategy, it is also prohibitively expensive and impacts both OpEx and CapEx for most organizations in labor, downtime, and implementation complexity.
This is where artificial intelligence, machine learning, and natural language processing can flip the paradigm, and achieve similar — and even enhanced — outcomes at a far lower cost. AI can be programmed to look for specific traits of behavior and identify quickly anomalies within industrial processes, claim submissions, and banking transactions, but with more consistent results and keener insights than a human counterpart.
Real-world use cases of AI Risk Management
Imagine if you could predict when a piece of machinery is due for replacement with over 90% accuracy, and do so before it actually fails. Or if you take immediate steps to prevent fraud by blocking credit card transactions automatically. Perhaps you could significantly reduce litigation costs by identifying cases through early intervention that trend towards litigation. This is all possible with AI.
Machine learning algorithms are capable of learning non-human programmed insights by analyzing data and discovering patterns that a typical human analyst might not. For instance, these insights could arise from changes in behavior in response to controls established within an insurance company, or may also be gleaned by identifying patterns in machinery that could point to an upcoming failure. They can also be driven by usage patterns in a credit card system that points to a fraudulent transaction.
The point is that there many, many applications of AI Risk Management and ML that can be applied across industries and verticals to improve outcomes and reduce the negative effects of different types of failure. If you’re looking to take advantage of these technologies to supercharge your business, there are a few steps you should consider.
Three steps to adopting AI risk management
To prepare your organization to adopt any new technology, the first thing you need to do is get your stakeholders on board. This entails clear communication of the initiative, how it will be implemented, and how the new technologies will directly impact your company’s business objectives. If you can bridge the gap between the technology’s intention and the real business results you can achieve, you will align your stakeholders with your cause.
The second step is to identify your key performance indicators, assess your current performance, and how you want AI Risk Management to move the needle. This entails zeroing in on specific numerical targets relevant to your business and setting a timeline with goals. Your KPIs will completely depend on the markers relevant to your business. An insurance company may look to reduce the total time spent on evaluating claims, while a manufacturer might look to dramatically reduce downtime caused by machinery failure.
Third, don’t forget that introducing a new technological initiative isn’t as simple as layering a fancy new tool or system over your existing operations. Remember that if your objective is to drive meaningful change that transforms your business, this will necessarily require a shift in your business model and all that goes along with that. Early and anticipatory communication with your internal teams is key to encouraging a positive culture, helping employees stay aware and informed, and priming receptivity so as to minimize pushback. Just as you need stakeholder buy-in at the top, you will want your organization to embrace the change from the bottom up.
Conclusion
Stay away from the hype that tends to accompany hot tech topics like AI. Don’t be quick to jump in with something simply because it’s new and bleeding edge — remember to stay focused on your true business objectives, and only invest in technologies that will help you achieve those goals.